Arc2Face一键整合包
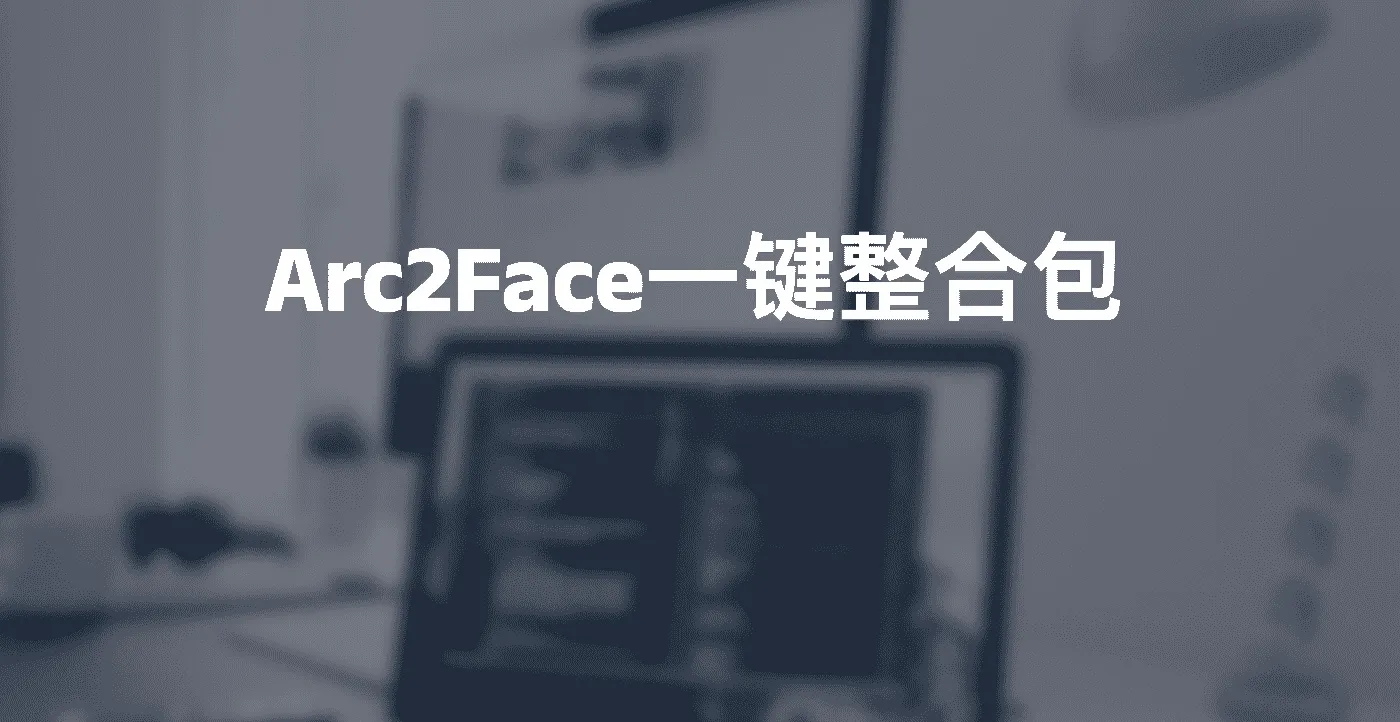
介绍
Arc2Face是用于人脸的基础模型训练,可批量生成超高质量主题的AI人脸艺术风格照,完美复制人脸
项目地址:https://github.com/foivospar/Arc2Face?tab=readme-ov-file#arc2face–controlnet-pose
特征
✅ 在大规模 WebFace42M 数据集上训练的几秒钟 ✅内,仅给定其 ArcFace 嵌入即可生成任何主题的高质量图像,与基于 Stable Diffusion 构建的现有模型 ✅相比,具有卓越的 ID 相似性,可以扩展到不同的输入模式,例如使用 ControlNet
整合包下载
夸克云盘: https://pan.quark.cn/s/6f1591a3dbfc
123云盘:https://www.123pan.com/s/9T6A-a9Qxd.html
演示
可前往:https://huggingface.co/spaces/FoivosPar/Arc2Face
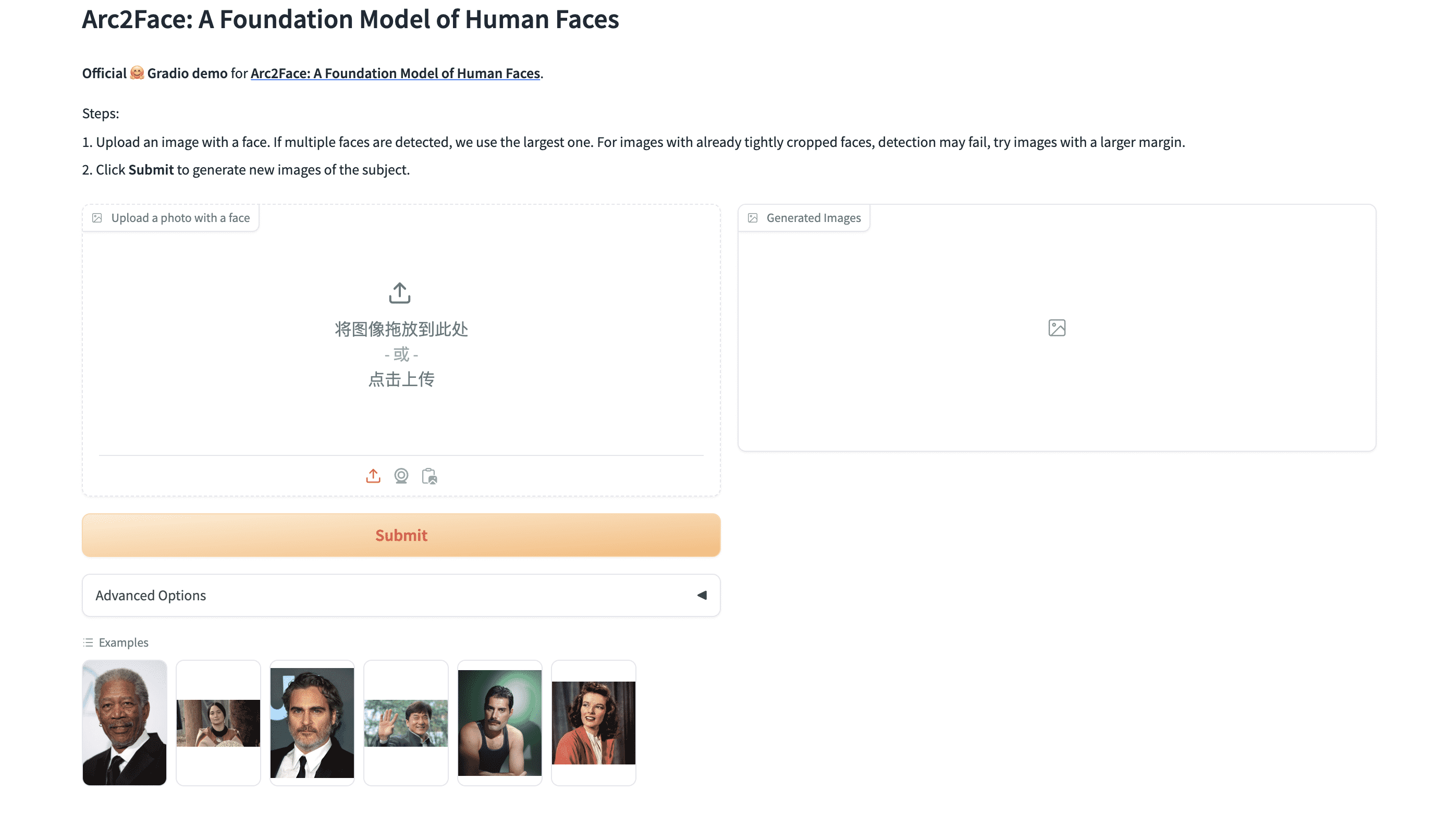
安装
conda create -n arc2face python=3.10
conda activate arc2face
# Install requirements
pip install -r requirements.txt
下载模型
- 可以从 HuggingFace 或使用 python 手动下载模型:
from huggingface_hub import hf_hub_download
hf_hub_download(repo_id="FoivosPar/Arc2Face", filename="arc2face/config.json", local_dir="./models")
hf_hub_download(repo_id="FoivosPar/Arc2Face", filename="arc2face/diffusion_pytorch_model.safetensors", local_dir="./models")
hf_hub_download(repo_id="FoivosPar/Arc2Face", filename="encoder/config.json", local_dir="./models")
hf_hub_download(repo_id="FoivosPar/Arc2Face", filename="encoder/pytorch_model.bin", local_dir="./models")
- 对于人脸检测和 ID 嵌入提取,请手动下载 antelopev2 包(直接链接)并将检查点放在 下。
models/antelopev2
- 我们使用在 WebFace42M 上训练的 ArcFace 识别模型。从 HuggingFace 下载并放入或使用 python:
arcface.onnx``models/antelopev2
hf_hub_download(repo_id="FoivosPar/Arc2Face", filename="arcface.onnx", local_dir="./models/antelopev2")
- 然后删除(insightface的默认主干)。
glintr100.onnx
文件夹结构最终应为:models
. ── models ──┌── antelopev2
├── arc2face
└── encoder
用法
使用(https://huggingface.co/docs/diffusers/index)加载:
from diffusers import (
StableDiffusionPipeline,
UNet2DConditionModel,
DPMSolverMultistepScheduler,
)
from arc2face import CLIPTextModelWrapper, project_face_embs
import torch
from insightface.app import FaceAnalysis
from PIL import Image
import numpy as np
base_model = 'runwayml/stable-diffusion-v1-5'
encoder = CLIPTextModelWrapper.from_pretrained(
'models', subfolder="encoder", torch_dtype=torch.float16
)
unet = UNet2DConditionModel.from_pretrained(
'models', subfolder="arc2face", torch_dtype=torch.float16
)
pipeline = StableDiffusionPipeline.from_pretrained(
base_model,
text_encoder=encoder,
unet=unet,
torch_dtype=torch.float16,
safety_checker=None
)
您可以使用任何兼容 SD 的调度程序和步骤,就像使用 Stable Diffusion 一样。默认情况下,我们使用 25 个步骤,只需几秒钟即可产生非常好的结果。DPMSolverMultistepScheduler
pipeline.scheduler = DPMSolverMultistepScheduler.from_config(pipeline.scheduler.config)
pipeline = pipeline.to('cuda')
选择一个图像并提取 ID 嵌入:
app = FaceAnalysis(name='antelopev2', root='./', providers=['CUDAExecutionProvider', 'CPUExecutionProvider'])
app.prepare(ctx_id=0, det_size=(640, 640))
img = np.array(Image.open('assets/examples/joacquin.png'))[:,:,::-1]
faces = app.get(img)
faces = sorted(faces, key=lambda x:(x['bbox'][2]-x['bbox'][0])*(x['bbox'][3]-x['bbox'][1]))[-1] # select largest face (if more than one detected)
id_emb = torch.tensor(faces['embedding'], dtype=torch.float16)[None].cuda()
id_emb = id_emb/torch.norm(id_emb, dim=1, keepdim=True) # normalize embedding
id_emb = project_face_embs(pipeline, id_emb) # pass through the encoder
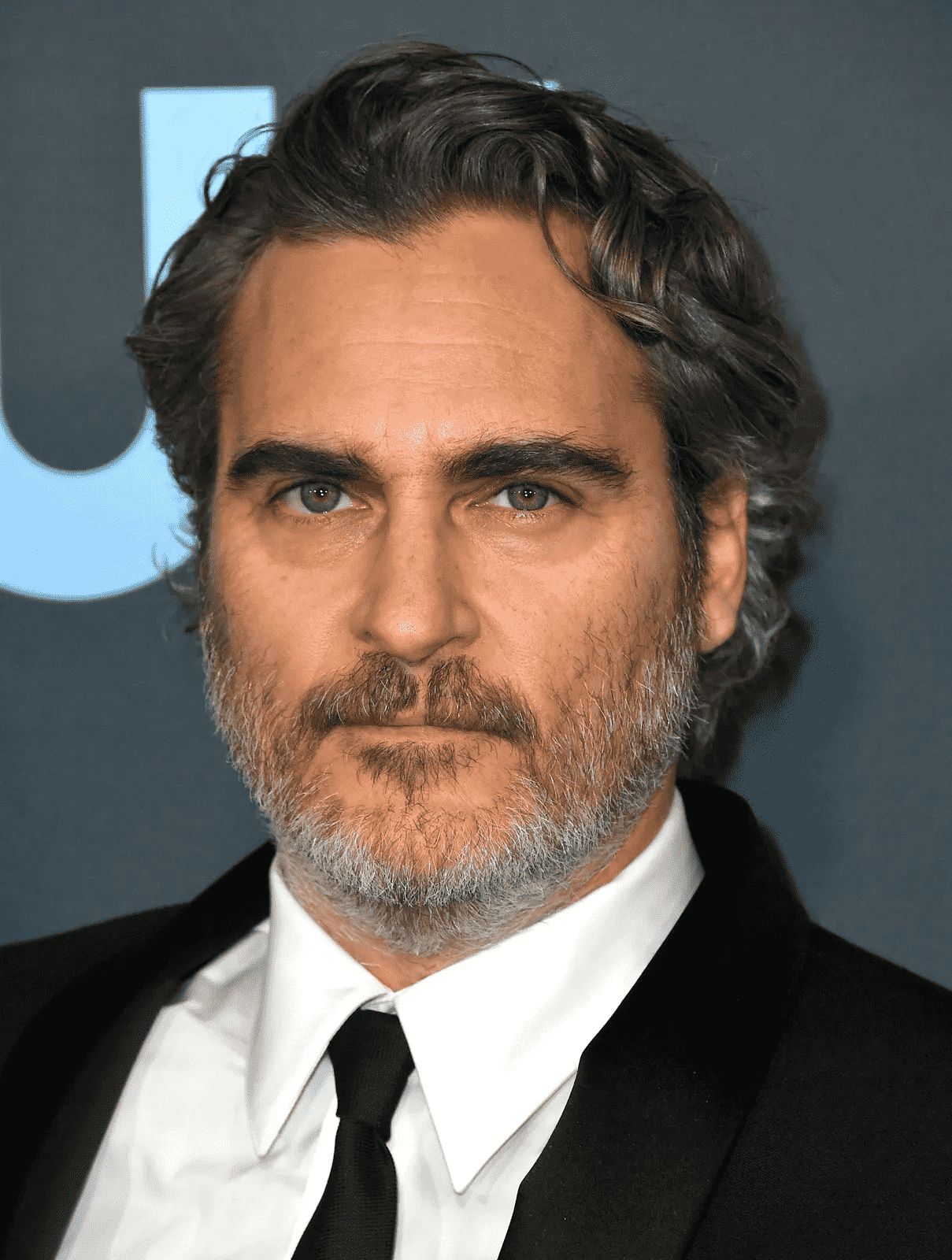
生成图像:
num_images = 4
images = pipeline(prompt_embeds=id_emb, num_inference_steps=25, guidance_scale=3.0, num_images_per_prompt=num_images).images
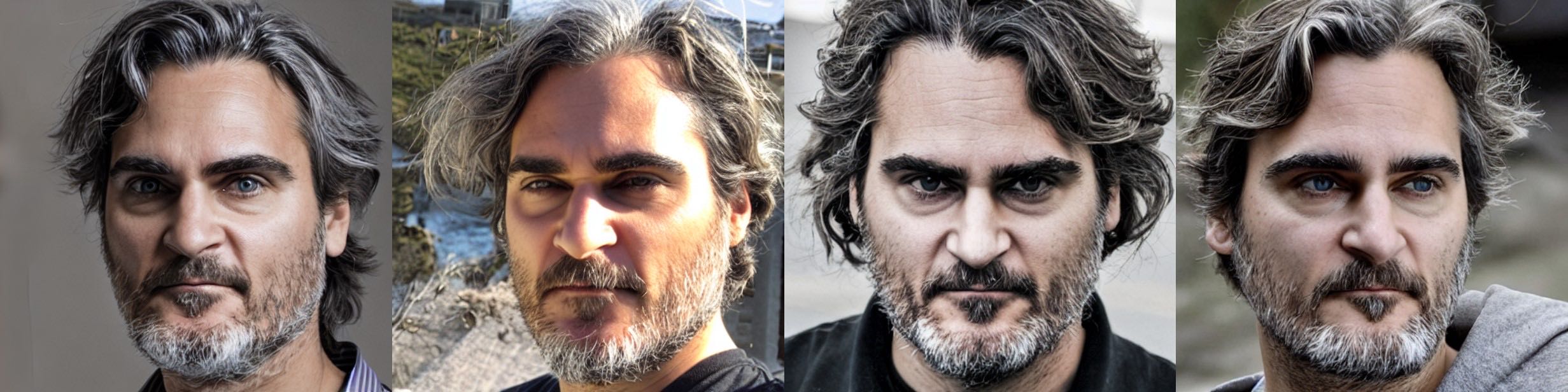
开始本地 gradio 演示
您可以通过运行以下命令来启动本地推理演示:
python gradio_demo/app.py
Arc2Face + ControlNet(姿势)
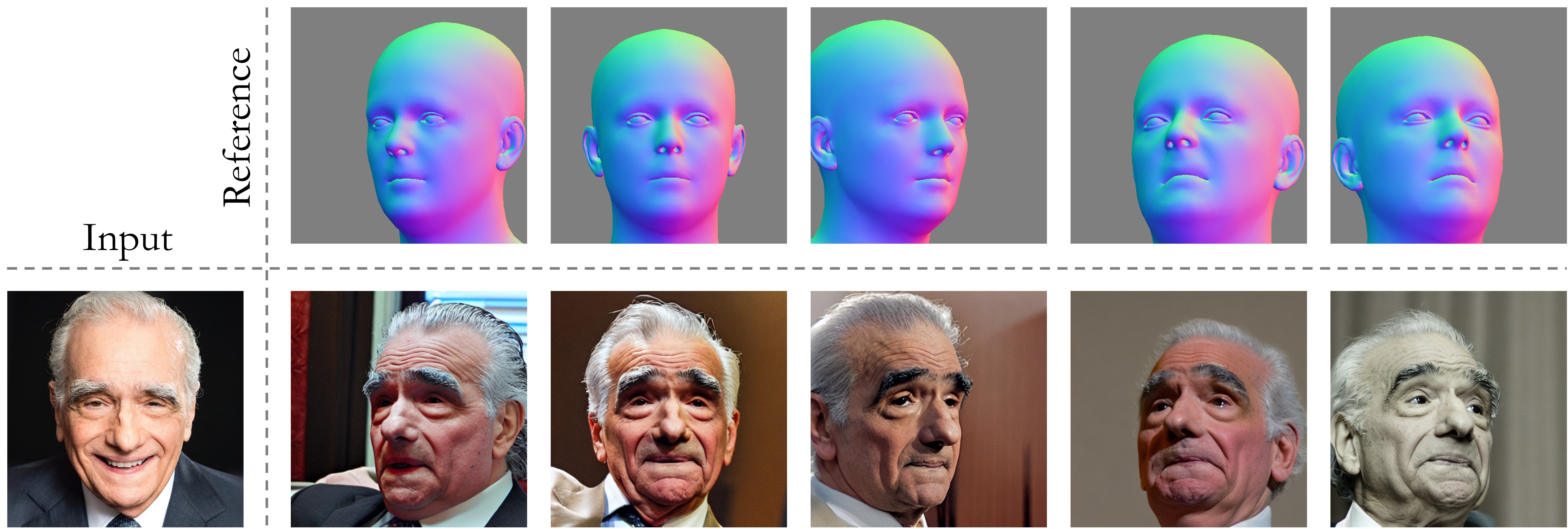
我们提供了一个在 Arc2Face 之上训练的 ControlNet 模型,用于姿势控制。我们使用 EMOCA 进行 3D 姿态提取。要运行我们的演示,请按照以下步骤操作:
1) 下载模型
从 HuggingFace 或使用 python 手动下载 ControlNet 检查点:
from huggingface_hub import hf_hub_download
hf_hub_download(repo_id="FoivosPar/Arc2Face", filename="controlnet/config.json", local_dir="./models")
hf_hub_download(repo_id="FoivosPar/Arc2Face", filename="controlnet/diffusion_pytorch_model.safetensors", local_dir="./models")
2) 拉动 EMOCA
git submodule update --init external/emoca
3) 安装
这是最棘手的部分。您将需要 PyTorch3D 来运行 EMOCA。由于其安装可能会导致冲突,我们建议遵循以下过程:
- 创建一个新环境,首先安装支持 GPU 的 PyTorch3D(按照官方说明进行操作)。
- 添加 Arc2Face + EMOCA 要求:
pip install -r requirements_controlnet.txt
- 安装 EMOCA 代码:
pip install -e external/emoca
- 最后,您需要下载 EMOCA/FLAME 资产。运行以下命令并按照终端中的说明进行操作:
cd external/emoca/gdl_apps/EMOCA/demos
bash download_assets.sh
cd ../../../../..
4) 启动本地 gradio 演示
您可以通过运行以下命令来启动本地 ControlNet 演示:
python gradio_demo/app_controlnet.py